Model for Speech Recognition Based on Multiple Time Scale Features
-
摘要: 提出了基于多时间尺度特征的语音识别模型.该模型采用描述谱参数轨迹的段特征,在段尺度上实现了对语音信号帧间相关性的显式建模;采用段特征依赖的非平稳时间序列产生模型,实现了不同尺度特征间的相关性建模,并在帧尺度上通过参数化的均值轨迹函数,实现了对语音信号帧间相关性的隐式建模.给出了基于多时间尺度特征联合统计距离优化的分段算法及基于最大似然准则的模型参数估计算法.识别实验表明,该模型的识别性能优于标准HMM及趋势HMM.Abstract: The model explicitly models the correlation among successive frames of speech signals in segment scale by using segmental features representing contours of spectral parameters. By using the proposed segmental feature dependent non-stationary time series model, the new model not only achieves the modeling of correlation between different scale features but also implicitly models the correlation among neighboring frames in frame scale via parametric mean trajectory function. A modified Viterbi algorithm based on joint statistical distance of multiple time scale features is proposed, and a algorithm based on the maximum likelihood criteria for estimating the model parameters is also proposed in the training. Experimental results show that the new model achieves better performance than the standard HMM and the trended HMM.
-
[1] Furui S. Speaker independent isolated word recognizer using dynamic features of speech spectrum[J]. IEEE Trans Acoust Speech Signal Process, 1981,34(1):52~59. [2]Deng L,Aksmanovic M,Sun D,et al.Speech recognition using hidden Markov models with polynomial regression functions as non-stationary states[J].IEEE Trans Speech Audio Processing,1993,2(4):507~520. [3]Juang B H,Rabiner L R.The segmental K-means algorithm for estimating parameters of hidden Markov models[J].IEEE Trans Acoust Speech Signal Process,1990,38(9):1639~1641. [4]Chen S H,Wang Y R.Vector quantization of pitch information in Mandarin speech[J].IEEE Trans Commun,1990,38:1317~1320. -
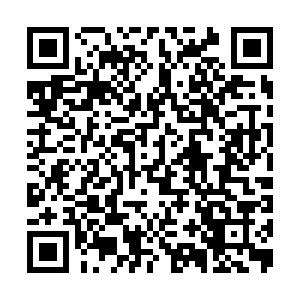
计量
- 文章访问数: 2732
- HTML全文浏览量: 61
- PDF下载量: 861
- 被引次数: 0