Relative position modification of SVM’s optimal hyperplane
-
摘要: 通过放宽标准支持向量机(SVM,Support Vector Machines)中类别边界至分类面等间隔的约束,保持两类函数间隔之和不变的条件,在支持向量机思想框架下得出分类面依样本分布进行调整的新型支持向量机,其对偶形式与标准支持向量机完全相同,从而在理论上进一步完善了支持向量机.在此基础上,提出使类别的函数间隔正比于样本标准差的具体算法——方差修正法,达到最优分类面的相对位置依样本方差而调整之目的.从统计意义上来说,方差修正法在分类精度上有所提高,但计算量增加不多.Abstract: Through releasing the equal-margin constraint in the standard support vector machines (SVM), keeping the sum of the binary-class function margins, a new SVM was gotten within the framework of SVM. The separating hyperplane of the new SVM can be adjusted as per the distribution of the binary-class samples, and its dual express is same as the standard SVM. Thus, the SVM was further improved theoretically. On the basis of the new SVM, a concrete algorithm, variance modification algorithm, was proposed. In the variance modification algorithm, the binary-class margins are in proportion to the standard deviation of binary-class samples. The goal of adjusting the optimal separating hyperplane as per sample-s variance is attained through the variance modification algorithm. Statistically, errors are reduced through the variance modification algorithm, while the computational complexity is not increased much.
-
Key words:
- support vector machines /
- modification /
- computational complexity /
- classifiers
-
[1] 边肇祺,张学工.模式识别[M].北京:清华大学出版社,2000 Bian Zhaoqi, Zhang Xuegong. Pattern recognition [M]. Beijing: Tsinghua University Press, 2000 (in Chinese) [2] Gert R G Lanckriet, Laurent El Ghaoui, Chiranjib Bhattacharyya, et al.A robust minimax approach to classification[J]. Journal of Machine Learning Research, 2002, 3:555-582 [3] Huang Kaizhu, Yang Haiqin, King Irwin, et al.Maxi-min margin machine: learning large margin classifiers locally and globally[J]. IEEE Transactions on Neural Networks, 2008,19(2): 260-272 [4] Wang Defeng, Yeung D S, Tsang E C C. Probabilistic large margin machine Proceeding of the Fifth International Conference on Machine learning and Cybernetics. Dalian: , 2006: 2190-2195 [5] Nello Cristianini, John Shawe-Taylor.支持向量机导轮[M]. 李国正,王猛,曾华军,译.北京:电子工业出版社,2004 Nello Cristianini, John Shawe-Taylor. An introduction to support vector machines and other kernel-based learning methods[M]. Translated by Li Guozheng, Wang Meng, Zeng Huajun. Beijing: Publishing House of Electronic Industry, 2004(in Chinese) [6] Vladimir N Vapnik. An overview of statistical learning theory[J]. IEEE Transactions on Neural Networks,1999, 10(5): 988-999 [7] 文传军,詹永照. 基于自调节分类面SVM 的平衡不平衡数据分类[J]. 系统工程, 2009,27(3):110-114 Wen Chuanjun, Zhan Yongzhao. Balance and imbalance data set classification based on self-adjusting classification-plane SVM[J]. Systems Engineering, 2009, 27(3):110-114(in Chinese) -
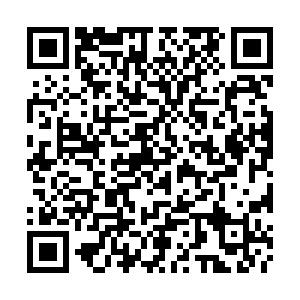
计量
- 文章访问数: 2496
- HTML全文浏览量: 90
- PDF下载量: 1044
- 被引次数: 0