Unsupervised classification approach based on graph-segment for multispectral remote sensing images
-
摘要: 针对传统基于像素的多光谱遥感图像分类方法存在的"麻点"现象、采样成本高等问题,提出了一种基于图论分割的非监督分类方法,首先采用基于图论的分割算法,按局部邻近相似像素点分割成若干子区域,再以分割后子区域为基本单元,整体进行模糊 C均值聚类,最终实现对多光谱图像的非监督分类.实验证明,该方法结合了局部邻近像素点的相互关系以及相似区域的整体特征,有效解决了麻点问题,具有较高的分类精度和算法效率,降低了采样成本.Abstract: To solving the noisy points and high cost problems of pixel-based multispectral image classification, a hybrid unsupervised approach with graph-based segment and fuzzy c-means clustering was presented. First, based on the relationships among neighboring pixels, image was segmented into groups of sub-regions using the graph-based algorithm. Then according to the global feature vector of sub-region, the fuzzy c-means classifier was used to obtain the classification map. Experiments turn out that the proposed approach, which considers both relationships of neighboring pixels and global feature of sub-region, can achieve better accuracy and efficiency by comparing the result with pixel-based fuzzy c-means classification.
-
[1] Agrawal A, Kumar N, Radhakrishna M. Multispectral image classification: a supervised neural computation approach based on rough-fuzzy membership function and weak fuzzy similarity relation [J]. International Journal of Remote Sensing, 2007, 28(20):4597-4608 [2] Yamazaki T, Gingras D. Unsupervised multispectral image classification using MRF models and VQ Method [J]. IEEE Transactions on Geoscience and Remote Sensing, 1999, 37(2):1173-1175 [3] Flygare A M. Classification of remotely sensed data utilizing the autocorrelation between spatial-temporal neighbors . Umea · : Department of Mathematical Statistics, Umea · University, 1997 [4] Yu Jun, Magnus Ekstrm. Multispectral image classification using wavelets: a simulation study [J]. Pattern Recognition, 2003, 36(4):889-898 [5] Lee J Y, Warner T A. Segment based image classification [J]. International Journal of Remote Sensing, 2006, 27(16):3403-3412 [6] Felzenszwalb P, Huttenlocher D. Efficient graph-based image segmentation [J]. International Journal of Computer Vision, 2004, 59(2):167-181 [7] Shi J, Malik J. Normalized cuts and image segmentation [J]. IEEE Transactions on Pattern Analysis and Machine Intelligence, 2000, 22(8):888-905 [8] Kanungo T, Mount D M, Netanyahu N S, et al. An efficient k-means clustering algorithm: analysis and implementation [J]. IEEE Transactions on Pattern Analysis and Machine Intelligence, 2002, 24(7):881-892 -
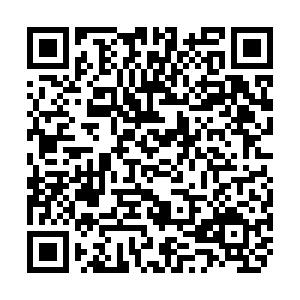
计量
- 文章访问数: 3185
- HTML全文浏览量: 200
- PDF下载量: 1079
- 被引次数: 0