Personalized interest modeling on portal based on latent interest semantic description
-
摘要: 日益丰富的门户个性化服务为兴趣建模提出了在描述以及扩展方面更高的要求.提出一种基于潜在兴趣语义描述的门户个性化兴趣模型(PIM-LISD,Personalization Interest Model based on Latent Interest Semantic Description),遵循有限混合模型理论,融入了隐式获取的门户个性化兴趣语义以及潜在兴趣语义关联描述.通过合理选取不同先验分布来适配潜在兴趣语义的后验分布,完善了模型的可解释性和自适应能力,并在建模过程中利用优化的期望最大化(TEM,Tempered Expectation Maximization)算法进行参数估计.实验表明该方法不仅有效节省建模开销,而且能够提升预测精度,从而验证了其正确性和有效性.Abstract: There is a great potential for interest modeling on description and extension as the personalization services enrich the portal. A novel personalization interest model based on latent interest semantic description(PIM-LISD) was proposed. As a finite mixture, it was represented with personalized interests elicited implicitly on portal and latent interest relating semantic descriptions. The initialization method was to fit the posterior probabilities along with the different reasonable prior predictions, so the interpretative and adaptive capacity of the model could be perfect. During the building procedure, an improved tempered expectation maximization (TEM) was used for variational expectation maximization estimation. The experiments indicate that the proposed modeling method can not only avoid the complication of potential expensive modeling-building stage effectively, but also increase the prediction precision, therefore proving its validity and feasibility.
-
[1] 张卫丰,徐宝文.基于WWW缓冲的用户实时二维兴趣模型[J].计算机学报, 2004,27(4):461-470 Zhang Weifeng, Xu Baowen. WWW cache based model of users’ real time two-dimensions interest[J]. Chinese Journal of Computers, 2004, 27(4): 461-470 (in Chinese) [2] Webb G, Pazzani M, Billsus D. Machine learning for user modeling[J]. User Modeling and User-Adapted Interaction, 2001, 11:19-29 [3] Hofmann T. Collaborative filtering via Gaussian probabilistic latent semantic analysis Proceedings of the ACM Conference on Research and Development in Information Retrieval. Canada:ACM Press, 2003:259-266 [4] Blei D, Ng A, Jordon M. Latent Dirichlet allocation[J]. Journal of Machine Learning Research,2003, 3:993-1022 [5] Marlin B. Modeling user rating profiles for collaborative filtering Proceedings of the 17th Annual Conference on Neural Information Processing Systems. Canada:MIT Press,2003:345-354 [6] McLachlan G J, Peel D. Finite mixture models[M]. New York:Wiley, 2000 [7] Wu Jing, Xiong Zhang. A portal-oriented personalized recomme- ndation using meta-recommender engine Proceedings of 2006 International Conference on Artificial Intelligence.Beijing:BUPT Press, 2006:570-576 [8] Wu Jing, Zhang Pin, Sheng Hao, et al. Mining personalization interest and navigation patterns on portal Proceedings of the 11th Pacific-Asia Conference on Knowledge Discovery and Data Mining, Lecture Notes in Artificial Intelligence. Berlin:Springer-Verlag Heidelberg,2007,4426:948-955 [9] Harper F, Li X, Chen Y, et al. An economic model of user rating in an online recommender system Proceedings of the 10th International Conference on User Modeling, Lecture Notes in Computer Science.Berlin:Springer-Verlag Heidelberg, 2005,3538:307-316 -
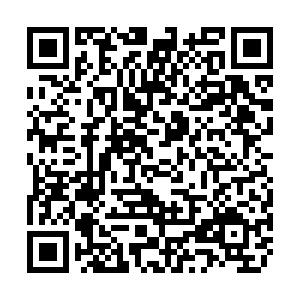
计量
- 文章访问数: 2635
- HTML全文浏览量: 121
- PDF下载量: 1194
- 被引次数: 0