-
摘要:
在计算稀疏主成分(PCs)时,由于同时求
k 个主成分的做法可以减少计算所产生的累积误差,因此提出了基于正则化秩k 矩阵逼近的稀疏主成分模型,并设计了求解该模型的块坐标下降法(BCD-sPCA-rSVD)。该算法的主要思想是先把变量按坐标分成2k 个块,当固定其他2k -1个坐标块的变量时,求解关于单个坐标块的子问题并给出子问题的显式解,循环地求解这些子问题直至满足终止条件。该算法每次迭代的计算复杂度关于样本个数与变量维数都是线性的,并且证明了它是收敛的。该算法不仅易于实现,数值仿真结果表明,该算法应用到真实数据与合成数据上都是可行且有效的。它不仅使累积误差降低,而且具有较低的计算复杂度,因而可以有效地求解大规模稀疏主成分分析问题。Abstract:In calculating the sparse principal components (PCs), attaining
k PCs simultaneously can reduce the accumulated error arising from the calculation process. We proposed the sparse principal component model via regularized rank-k matrix approximation and designed a block coordinate descent method (BCD-sPCA-rSVD) to solve this problem. Its main idea is to first divide variables into 2k blocks by coordinates, and then solve sub-problem with respect to each single coordinate block when keeping other 2k -1 variables fixed. By solving these sub-problems with explicit solutions recursively until the stopping criterion is satisfied, the BCD-sPCA-rSVD algorithm can be easily constructed. Its per-iteration complexity is linear in both sample size and variable dimensionality. The algorithm is convergent and easy to implement. Numerical simulation results show that the algorithm is feasible and effective when applied to real and synthetic data sets. The proposed method reduces the accumulated error and has lower computational complexity, which makes it well suited to handling large-scale problems of sparse principal component analysis. -
表 1 各稀疏PCA算法的计算复杂度
Table 1. Computational complexity of each sparse PCA algorithm
算法 计算复杂度 n>p n<p BCD-sPCA-rSVD O(Jk+nk2) O(Jk+ pk2) SPCA O(p3) O(Jk+ pk2) BCD-SPCA O(Jk+nk2) O(Jk+ pk2) 表 2 Pitprop数据:各稀疏PCA算法的性能指标
Table 2. Pitprop data: Performance indicators of each sparse PCA algorithm
算法 稀疏度 非正交性 相关性 PEV/% RRE/% PCA 0 0 0 86.94 36.06 DSPCA 63 13.63 0.57 72.46 47.71 Gpower 63 17.88 0.51 75.04 45.89 SCoTLASS 27 0.32 0.44 78.24 49.24 ALSPCA 63 0 0.30 73.32 45.37 sPCA-rSVD-soft 53 14.76 0.46 76.59 46.76 SPCA 60 0.86 0.40 75.82 44.48 BCD-SPCA 63 20.05 0.40 75.86 44.19 BCD-sPCA-rSVD 63 1.51 0.28 75.13 44.18 表 3 合成数据:PCA与各稀疏PCA算法的载荷
Table 3. Synthetic data: Loadings of PCA and each sparse PCA algorithm
变量 PCA BCD-sPCA-rSVD SPCA(BCD-SPCA) PC1 PC2 PC1 PC2 PC1 PC2 X1 -0.116 3 -0.478 4 0 -0.500 0 0 -0.500 0 X2 -0.116 2 -0.478 4 0 -0.500 0 0 -0.500 0 X3 -0.116 2 -0.478 4 0 -0.500 0 0 -0.500 0 X4 -0.116 2 -0.478 3 0 -0.500 0 0 -0.500 0 X5 0.395 1 -0.145 3 0.500 0 0 0.500 0 0 X6 0.395 1 -0.145 3 0.500 0 0 0.500 0 0 X7 0.395 1 -0.145 4 0.500 0 0 0.500 0 0 X8 0.395 1 -0.145 3 0.500 0 0 0.500 0 0 X9 0.400 9 0.009 1 0 0 0 0 X10 0.400 9 0.0091 0 0 0 0 PEV/% 99.72 80.46 80.46 表 4 Colon Cancer数据:各稀疏PCA算法的性能和效率指标
Table 4. Colon Cancer data: Performance and efficiency indicators of each sparse PCA algorithm
算法 稀疏度 非正
交性相关性 PEV/
%RRE/
%计算
时间/
s迭代
次数PCA 0 0 0 58.35 64.54 1.98 SPCA 5 370 22.88 0.49 46.12 65.48 4.47 165 BCD-
SPCA5 373 29.15 0.54 48.88 69.73 3.65 97 BCD-sPCA-
rSVD5 376 24.02 0.48 47.24 65.34 2.76 91 表 5 20Newsgroups数据:各稀疏PCA算法的性能和效率指标
Table 5. 20Newsgroups data: Performance and efficiency indicators of each sparse PCA algorithm
算法 稀疏度 非正
交性相关性 PEV/
%RRE/
%时间/
s迭代
次数PCA 0 0 0 10.69 94.50 1.08 SPCA 161 0.07 0.15 8.42 95.76 2.62 192 BCD-SPCA 161 17.64 0.30 8.71 95.34 3.11 58 BCD-sPCA-
rSVD166 0.02 0.09 8.58 95.60 2.73 52 -
[1] ZOU H, HASTIE T.Sparse principal component analysis[J].Journal of Computational and Graphical Statistics, 2006, 15(2):265-286. doi: 10.1198/106186006X113430 [2] TRENDAFILOV N T, JOLLIFFE I T.Projected gradient approach to the numerical solution of the SCoTLASS[J].Computational Statistics and Data Analysis, 2006, 50(1):242-253. doi: 10.1016/j.csda.2004.07.017 [3] D'ASPREMONT A, GHAOUI L E, JORDAN M I, et al.A direct formulation for sparse PCA using semidefinite programming[J].SIAM Review, 2007, 48(3):434-448. http://papers.nips.cc/paper/2628-a-direct-formulation-for-sparse-pca-using-semidefinite-programming.pdf [4] SHEN H, HUANG J Z.Sparse principal component analysis via regularized low rank matrix approximation[J].Journal of Multivariate Analysis, 2008, 99(6):1015-1034. doi: 10.1016/j.jmva.2007.06.007 [5] MOGHADDAM B, WEISS Y, AVIDAN S.Spectral bounds for sparse PCA:Exact and greedy algorithms[C]//Advances in Neural Information Processing Systems.Montreal:Neural Information Processing System Foundation, 2006:915-922. [6] D'ASPREMONT A, BACH F R, GHAOUI L E.Optimal solutions for sparse principal component analysis[J].Machine Learning, 2008, 9(7):1269-1294. https://www.researchgate.net/publication/1897313_Optimal_Solutions_for_Sparse_Principal_Component_Analysis [7] LUSS R, TEBOULLE M.Conditional gradient algorithms for rank-one matrix approximations with a sparsity constraint[J].SIAM Review, 2013, 55(1):65-98. doi: 10.1137/110839072 [8] LUSS R, TEBOULLE M.Convex approximations to sparse PCA via Lagrangian duality[J].Operations Research Letters, 2011, 39(1):57-61. doi: 10.1016/j.orl.2010.11.005 [9] SIGG C, BUHMANN J.Expectation-maximization for sparse and nonnegative PCA[C]//Proceedings of the 25th International Conference on Machine Learning.NewYork:ACM, 2008:960-967. [10] JOURNEE M, NESTEROV Y, RICHTARIK P, et al.Generalized power method for sparse principal component analysis[J].Journal of Machine Learning Research, 2010, 11(2):517-553. http://www.oalib.com/paper/3867039 [11] LU Z S, ZHANG Y.An augmented Lagrangian approach for sparse principal component analysis[J].Math Program Series A, 2012, 135(1-2):149-193. doi: 10.1007/s10107-011-0452-4 [12] ZHAO Q, MENG D Y, XU Z B, et al.A block coordinates descent approach for sparse principal component analysis[J].Neurocomputing, 2015, 153(4):180-190. http://gr.xjtu.edu.cn/c/document_library/get_file?folderId=1834195&name=DLFE-34834.pdf [13] TIBSHIRANI R. Regression shrinkage and selection via the LASSO[J].Journal of the Royal Statistical Society Series B, 1996, 58(3):267-268. http://citeseerx.ist.psu.edu/viewdoc/download?doi=10.1.1.35.7574&rep=rep1&type=pdf [14] TSENG P.Convergence of a block coordinates descent method for nondifferentiable minimization[J].Journal of Optimization Theory Apply, 2001, 109(3):475-494. doi: 10.1023/A:1017501703105 [15] JEFFERS J N R.Two case studies in the application of principal component analysis[J].Applied Statistics, 1967, 16(3):225-236. doi: 10.2307/2985919 [16] ALON U, BARKAI N, NOTTERMAN D A, et al.Broad patterns of gene expression revealed by clustering of tumor and normal colon tissues probed by oligonucleotide arrays[J].Proceedings of the National Academy of Sciences of the United States of America, 1999, 96(12):6745-6750. doi: 10.1073/pnas.96.12.6745 -
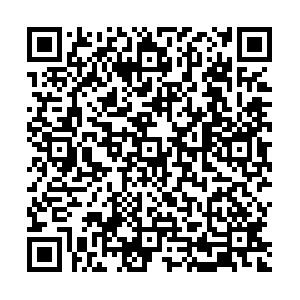
计量
- 文章访问数: 567
- HTML全文浏览量: 138
- PDF下载量: 5
- 被引次数: 0