Identification of Optimal Subspace from PLS Regression
-
摘要: 偏最小二乘回归是一种新型的多元分析方法.它可以在自变量多重相关的条件下,有效地构造出对系统解释性最强的子空间,进行回归建模,使模型的精度和可靠性得到很大的提高.本文提出采用因素分析方法,对偏最小二乘回归的最优子空间进行正交变换.这种变换方法对偏最小二乘回归的模型结果没有任何影响,却可以使最优子空间的实际含义得到更好的解释.案例研究表明,经过正交变换后,原始变量被分为若干变量组,每个变量组分别对应于最优子空间中的一个因素,从而提高了对最优子空间的内涵分析能力.Abstract: Partial least-squares regression, a novel approach for multivariate analysis, is widely used for modeling a multi-collinear variable data set, with improved model accuracy and reliability based on building a subspace with most explanatory ability to the data set. In this paper the factor analysis method is presented to transform orthogonally the optimal subspace, which is obtained from partial least-squares regression. The transformation can identify each factor in a meaningful way but does not change the results of partial least-squares regression model. Therefore, the physical meaning of the optimal subspace of partial least-squares regression can be illustrated. A case study demonstrates that the original variable set is divided into several variable groups after the orthogonal transform, each of which is corresponding to a new factor in the subspace such that its explanatory ability is improved.
-
Key words:
- subspaces /
- explanation /
- factor analysis /
- partial least-squares regression /
- simple structure
-
[1] Tenenhaus M. La regression PLS theorie et pratique[M]. Paris:Editions Technip, 1998. [2]Wold S, Ruhe A, Wold H, et al. The collinearity problem in linear regression, The partial least squares (PLS) approach to generalized inerses[J]. SIAM J Science Statistics Computer, 1984,5(2):735~743. [3]Wold H. Partial least squares, in encyclopedis of statistical sciences[M]. New York:John Wiley & Ston, 1985, 581~591 [4]王惠文. 偏最小二乘回归方法以及应用[M]. 北京:国防工业出版社,1999. [5]Tenenhaus M, Gauchi J P, Menardo C. Regression PLS et applications[J]. Revue de Statistique Appliquee, 1995,53(1):7~63. [6]王 权. 现代因素分析[M]. 杭州:杭州大学出版社,1992. -
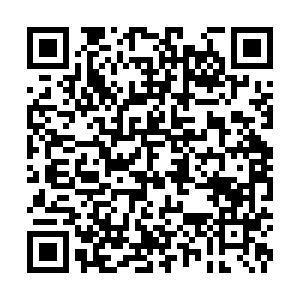
计量
- 文章访问数: 585
- HTML全文浏览量: 51
- PDF下载量: 507
- 被引次数: 0