Two-layer clustering over data stream with fault-tolerance
-
摘要: 提出一种具有容错能力的进化数据流聚类算法FTGDStream (Fault-Tolerant Grid-Density Clustering over Data Stream),通过在聚类过程中引入适当的松弛条件,从而在含有噪声的真实世界数据中获取更加泛化的有用知识.首先利用基于相似性度量和小波技术的HLSFTS (Hierarchical Lifting Scheme Fault-Tolerant Synopses)层次概要数据结构实现在线微聚类过程,然后采用基于网格密度的聚类算法实现离线宏聚类过程.在线算法所构造的小波概要数据结构对原始数据的高压缩率降低了离线网格密度聚类算法的计算负载,提高了二层数据流聚类算法的效率.在UCI数据集上的仿真实验结果表明,FTGDStream算法可以聚类任意空间形状的数据并且适用于高维数据流环境,是一种具有容错能力的高效数据流聚类算法.Abstract: A new envolving data stream clustering algorithm with fault-tolerance characteristic was proposed named FTGDStream (fault-tolerant grid-density clustering over data stream). It introduces appropriate relaxation of conditions for discover generalised knowledge in real world data polluted by noise. First, FTGDStream uses similarity measure technology and lifting wavelet to construct synopsis HLSFTS (hierarchical lifting scheme fault-tolerant synopses) to realize online micro-cluster phase. Second, FTGDStream uses grid-density clustering technology to realize offline macro-cluster phase. High compression ratio of HLSFTS in micro-cluster reduces the computation load of grid-density clustering algorithm in macro-cluster and improves the efficiency of two-layer algorithm. Simulation in UCI data set proves that FTGDStream is able to clustering any shape in data space and suitable for dealing with high-dimensional data streams. FTGDStream is an efficient clustering algorithm with fault-tolerance.
-
Key words:
- evolving data stream clustering /
- fault-tolerance /
- synopses /
- grid density
-
[1] Callaghan O L,Mishra N,Meyerson A.Streaming data algorithms for high-quality clustering[C]//San Jose.Proceedings of International Conference on Data Engineering.California:IEEE Computer Society,2002:685-699 [2] Chen Y X,Tu L.Density-based clustering for real-time stream data[C]//San Jose.Proceedings of the 13th ACM SIGKDD International Conference on Knowledge Discovery and Data Mining.California:ACM,2007:133-142[ZK)] [3] Aggarwal C C,Han Jiawei,Wang Jianyong,et al.A framework for clustering evolving data streams[C]//Proceeding of the 29th International Conference on Very Large Data Bases.Berlin:Morgan Kaufmann ,2003:81-92 [4] Aggarwal C C,Han Jiawei,Wang Jianyong,et al.A framework for projected clustering of high dimensional data streams[C]//Proceeding of the 29th International Conference on Very Large Data Bases.Toronto,Canada:Morgan Kaufmann,2004:852-863 [5] Chen Mingsheng,Wu Xianliang,Wei Sha,et al.Fast multipole method accelerated by lifting wavelet transform scheme[J].Applied Computational Electromagnetics Society Journal,2009,24(2):109-115 [6] Shahin M,Badawi A,Kamel M.Biometric authentication using fast correlation of near infrared hand vein patterns [J].International Journal of Biometrical Sciences,2007,2 (3):141-148 [7] Cao Feng,Ester M,Qian Weining,et al.Density-based clustering over an evolving data stream with noise[C]//Proceedings of the 6th SIAM International Conference on Data Mining.Bethesda,MD:SIAM,2006:326-337 [8] Han J,Kamber M.Data mining:concepts and techniques[M].2nd ed.Morgan Kaufmann:Elsevier Inc,2006:467-589 -
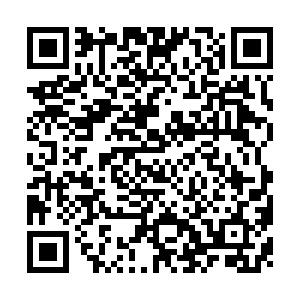
计量
- 文章访问数: 2441
- HTML全文浏览量: 200
- PDF下载量: 436
- 被引次数: 0