Adaptive subspace band selection method based on spectrum characteristics
-
摘要: 针对现有波段选择方法无法依据感兴趣地物划分数据子空间及子空间划分容易受背景地物影响等问题,提出一种基于光谱特征的自适应子空间波段选择方法.利用感兴趣地物的光谱曲线,建立光谱自适应因子(SAF,Spectral Adaptive Factor);通过聚类划分子空间,在每个子空间内,利用J-M距离筛选类别可分性最大波段作为子空间最优波段,从而得到整个数据空间的最佳波段组合.利用AOTF (Acousto-Optic Tunable Filter)成像光谱仪数据与波段指数(BI,Band Index)和基于类间可分性最佳波段选择两种方法比较,SAF方法选择的最佳波段组合,包含信息量丰富,地物之间差异较大,且地物平均J-M距离大于另外两种方法.用最大似然法(maximum likelihood)对最佳波段组合进行分类,总体分类精度为96.8%,Kappa系数为0.894.结果验证了SAF方法的有效性和实用性.Abstract: Adaptive subspace band selection method based on spectrum characteristics was proposed to solve the problems, including the existing methods which couldn't divide subspace by studied features and background features easily affected the result of subspace division.Spectral adaptive factor (SAF) was established with the spectral curves of studied features, and the whole data space was divided into some subspace based on clustering. In each subspace, Jeffreys-Matusita distance was calculated to choose the maximum class separability band as the optimal band. The optimal bands combination was achieved.With the data of acousto-optic tunable filter (AOTF) imaging spectrometer,an experiment was accomplished to compare with other band selection methods, involving band index (BI) method and the optimal bands selection method based on the classes distinguish ability. Experimental results show that the optimal bands combination of the proposed method contains better performance and studied features are shown more significant difference. And the average of Jeffreys-Matusitadistance of all classes of the proposed method is the greatest of all methods.Maximum likelihood classification method was also implemented on the images of the optimal bands combination of the proposed method. As a result, the overall accuracy is 96.8% and Kappa coefficient is 0.89.The experiment indicates that the proposed method is effectiveness and practicability.
-
Key words:
- hyperspectral remote sensing /
- band selection /
- subspace partition
-
[1] 童庆禧,张兵,郑兰芬.高光谱遥感——原理、技术与应用[M].北京:高等教育出版社,2006:1-3
Tong Qingxi,Zhang Bing,Zheng Lanfen.Hyperspectral remote sensing:the principle,technology and application[M].Beijing:Higher Education Press,2006:1-3(in Chinese)[2] 韩瑞梅,杨敏华.一种改进的高光谱遥感数据波段选择方法的研究[J].测绘与空间地理信息,2010,33(3):137-139
Han Ruimei,Yang Minhua.Study on an improved method of band selection of hyperspectral remote sensing data[J].Geomatics & Spatial Information Technology,2010,33(3):137-139(in Chinese)[3] 苏红军,盛业华.基于正交投影散度的高光谱遥感波段选择算法研究[J].光谱学与光谱分析,2011,31(5):1309-1313
Su Hongjun,Sheng Yehua.Orthogonal projection divergence based hyperspectral band selection[J].Spectroscopy and Spectral Analysis,2011,31(5):1309-1313(in Chinese)[4] Huang Rui,He Mingyi.Band selection based on feature weighting for classification of hyperspectral data[J].IEEE Geoscience and Remote Sensing Letter,2005,2(2):156-159 [5] Bajcsy P,Groves P.Methodology for hyperspectral band selection[J].Photogrammetric Engineering and Remote Sensing Journal,2004,70(7):793-802 [6] 刘春红.超光谱遥感图像降维及分类方法研究[D].哈尔滨:哈尔滨工程大学信息与通信学院,2005
Liu Chunhong.Research on dimensional reduction and classification of hyperspectral remote sensing image[D].Harbin:College of Signal and Information Processing,Harbin Engineering University,2005(in Chinese)[7] 张良培,张立福.高光谱遥感[M].北京:测绘出版社,2011:83-87
Zhang Liangpei,Zhang Lifu.Hyperspectral remote sensing[M].Beijing:Surveying and Mapping Press,2011:83-87(in Chinese)[8] 齐腊,刘良云,赵春江,等.基于遥感影像时间序列的冬小麦种植监测最佳时相选择研究[J].遥感技术与应用,2008,23(2):154-160
Qi La,Liu Liangyun,Zhao Chunjiang,et al.Selection of optimum periods for extracting winter wheat based on multi-temporal remote sensing images[J].Remote Sensing Technology and Application,2008,23(2):154-160 (in Chinese)[9] 孙丽娟.基于支持向量机的高光谱图像分类技术研究[D].哈尔滨:哈尔滨工程大学信息与通信学院,2011
Sun Lijuan.Research on classification of hyperspectral images based on support vector machin[D].Harbin:College of Signal and Information Processing,Harbin Engineering University,2011(in Chinese) -
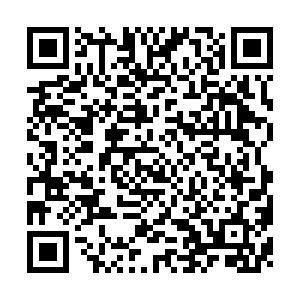
计量
- 文章访问数: 1491
- HTML全文浏览量: 46
- PDF下载量: 738
- 被引次数: 0