Learning drifting user interest incrementally from numerically labeled feedbacks
-
摘要: 针对现有兴趣漂移增量学习方法大多针对包含二值数据标签的用户反馈进行学习的不足,提出了一种适用于连续数值标签反馈的兴趣漂移增量学习方法,以或然概念集的形式描述用户兴趣,将用户反馈中包含的数据标签视为用户对该实例的喜好概率,并采用基于指数近度加权平均的方法对兴趣模型进行增量学习.在不同学习任务下的实验结果表明,该方法能够在反馈数据标签为连续数值的条件下达到比现有方法更好的学习效果.Abstract: Most incremental approaches for learning drifting user interests assume that data instances in user feedbacks are binary labeled. A novel incremental learning approach was presented which learns drifting user interests from numerically labeled feedbacks instead of binary labeled ones. User interests were modeled as a set of probabilistic concepts. Numerical instance labels were considered as probabilities that the user likes those instances. Feedbacks were used to update user interest models incrementally based on an exponential, recency-weighted average algorithm. Experimental results on different learning tasks showed that the approach outperforms existing approaches in numerically labeled feedback environment.
-
Key words:
- learning algorithms /
- reinforcement learning /
- fuzzy sets
-
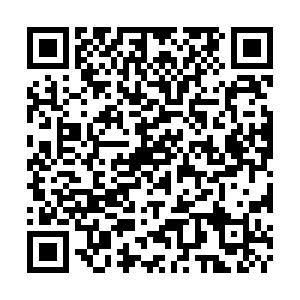
计量
- 文章访问数: 3262
- HTML全文浏览量: 113
- PDF下载量: 1161
- 被引次数: 0