Fault prognostic algorithm based on dual estimation and particle filter
-
摘要: 假设对象系统的故障演化过程可以由一个含有未知缓变参数的状态空间模型加以描述,则故障预测问题就可以转化为一个在已知当前系统信息的条件下,对系统未来某一时刻的状态变量的估计问题.针对该问题的求解提出了一种基于二元估计和粒子滤波的故障预测算法.算法的实施分为两个主要阶段:在状态估计阶段,采用两个并联的粒子滤波器迭代估计当前时刻对象系统故障演化模型状态和未知参数的后验分布.在状态预测阶段,对当前时刻故障演化模型状态的后验分布进行迭代采样,以采样样本粒子来近似估计未来时刻的状态变量的先验分布密度.在上述计算结果的基础上,结合相应的故障判据,算法采用计算对象系统未来时刻故障概率的方法预测其剩余使用寿命.仿真实验中将本文提出的算法与基于联合估计的故障预测算法进行对比,实验结果证明了所提算法的有效性.Abstract: Supposing that system fault evolution process is expressed by a state space model with unknown slow time-variant parameters, then the fault prognostics can be formulated as a problem of estimating model states for some future time while knowing all the information about system till time step now. An algorithm based on dual estimation and particle filter was presented. This algorithm includes two major stages:on the state estimation stage, it used an iterative procedure to estimate the posterior distributions of system states and parameters alternatively based on two parallel connected particle filters; on the state prediction stage, the algorithm sampled former estimated posterior distributions iteratively and used the sampled particles to form the prior distributions of system states for some future time. Based upon above calculated results and combined with certain fault criterions, the time to failure could then be inferred by computing the probability of system failure. Comparing with the joint estimation approach, the simulation result demonstrates the validity and feasibility of proposed algorithm.
-
Key words:
- fault prognostics /
- particle filter /
- joint estimation /
- dual estimation
-
[1] Ray A, Tangirala S. Stochastic modeling of fatigue crack dynamics for on-line failure prognostics[J]. IEEE Transactions on Control Systems Technology, 1996, 4(4):443-451 [2] Swanson D C. A general prognostic tracking algorithm for predictive maintenance Proceedings of IEEE Aerospace Conference. Big Sky, Mantana:IEEE, 2001:2971-2977 [3] Chelidze D. Multimode damage tracking and failure prognosis in electromechanical system Willett P K, Kirubarajan T. Components and Systems Diagnostics, Prognostics, and Health Management II, Proceedings of SPIE. Orlando:SPIE, 2002:1-12 [4] Wan E A, Van Der Merwe R. The unscented Kalman filter for nonlinear estimation Adaptive Systems for Signal Processing, Communication, and Control Symposium 2000. Lake Louise, Alta:IEEE, 2000:153-158 [5] Doucet A, Godsill S J, Andrieu C. On sequential Monte Carlo sampling methods for Bayesian filtering[J]. Statistics and Computing, 2000, 10(3):197-208 [6] Xie X Q, Zhou D H, Jin Y H. Strong tracking filter based adaptive generic model control[J]. Journal of Process Control, 1999, 9(4):337-350 -
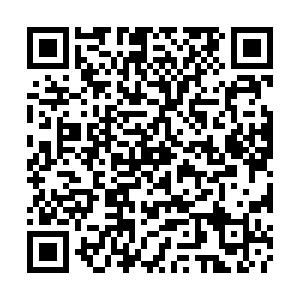
计量
- 文章访问数: 3188
- HTML全文浏览量: 130
- PDF下载量: 966
- 被引次数: 0