Modeling strategy of principle component regression
-
摘要: 分析了国际上通用的主成分回归的工作原理和失效原因.在此基础上,提出一种新的主成分回归建模策略:①提取所有主成分建立模型;②删除模型中t检验不显著的成分;③用t检验显著的成分建立最终需要的模型.由于任一主成分的回归系数和t检验值以及与其余主成分无关.因此,当采用向后删除变量法时,如果有多个成分t检验不显著,则可以将它们同时删除,而无须逐个删除.采用仿真案例对所提出的方法的合理性进行验证.这种新的建模策略可以有效地提取对因变量有较强解释作用的成分,实现在自变量多重相关条件下的回归建模,并且允许在模型中包含所有的原始变量.此外,该方法的成分筛选过程简便,累计计算误差小于偏最小二乘回归等迭代算法.Abstract: When the mechanism and the reason of failure of the classical principal components regression were analyzed, a new strategy of PCR modeling was presented as:①deriving all components and modeling with all these components; ②exclude all components which were not significant in t-test; ③modeling with the components which were significant in t-test. Proved the regression coefficient and the t-test value of any principal component were unrelated to the other principal components. It was insured that, when applying backward-delete variables law, all the variables which were not significant in t-test test could be deleted together at the same time. It was not necessary to delete them gradually. A simulation study was given to prove the validity of the strategy. The research indicates that the suggested strategy can effectively derive components which are explainable to dependent variables. Modeling under the condition of multicollinearity is enabled, and all the independent variables can be included. The process of suggested variables selection method is simple, and the accumulated error is smaller than that of partial least-squares regression.
-
Key words:
- regression analysis /
- principal component analysis /
- components /
- selection
-
[1] Hoerl A E, Kennard R W. Ridge regression:biased estimation for non-orthogonal problems[J]. Teehno Metrics, 1970, 12:55-68 [2] Hoerl A E, Kennard R W. Ridge regression:application for non-orthogonal problems[J]. Teehno Metrics, 1970, 12:69-72 [3] Wold S, Albano C, Dunn M, et al. Pattern regression finding and using regularities in multivariate data[M]. London:Analysis Applied Science Publication, 1983 [4] Wold S, Martens H, Wold H. The multivariate calibration problem in chemistry solved by the PLS method Ruhe A, K gstr m B. Proc Conf Matrix Pencils Lectures Notes in Mathematics. Heidelberg:Springer-Verlag, 1983 [5] Tenenhaus M, L-approche P L S. Revue de Statistique Appliqu e[M]. Paris :Springer-Verlag,1999 [6] Kutner, Nachtsheim, Neter. Applied linear regression models[M]. Fourth Edition. New York:McGraw-Hill,2005 [7] 王惠文.PLSR方法及其应用[M].北京:国防工业出版社,1999 Wang Huiwen. Partial least-squares regression method and application [M]. Beijing:National Defence Industry Press,1999(in Chinese) [8] Ergon R. Reduced PCR/PLSR models by subspace projections[J]. Chemometrics and Intelligent Laboratory Systems, 2006, 81:68-73 [9] Bjrn-Helge M,Henrik R C. Mean squared error of prediction (MSEP) estimates for principal component regression (PCR) and partial least squares regression (PLSR) [J].Journol of Chemometrics, 2004, 18:422-429 [10] Ergon R. Constrained numerical optimization of PCR/PLSR predictors [J]. Chemometrics and Intelligent Laboratory Systems, 2003, 65:293-303 [11] 任若恩,王惠文.多元统计数据分析——理论、方法、实例[M]. 北京:国防工业出版社, 1997 Ren Ruoen, Wang Huiwen. Statistical analysis on multivariate data-theories, methods, case studies[M]. Beijing:National Defence Industry Press, 1997(in Chinese) -
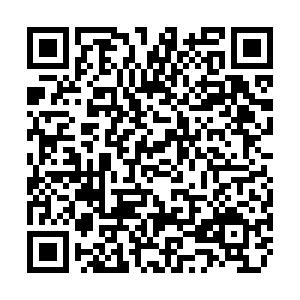
计量
- 文章访问数: 12051
- HTML全文浏览量: 182
- PDF下载量: 3958
- 被引次数: 0