Prediction modeling based on Bayes support vector machine
-
摘要: 对实际统计数据中存在的相关性、不确定性和非线性问题,提出贝叶斯支持向量机预测模型方法.构建基于高斯分布的权值分布模型描述信息的不确定性,基于先验概率分布和贝叶斯关系获得后验分布模型,利用极大似然方法和递推迭代算法求解后验分布的最佳参数,从而得到关联向量机.建立起基于参数分布多维时间序列预测模型,将每一步迭代过程中的支持向量机输入作为随机变量,考虑数据不确定性的传递,递推得到贝叶斯支持向量机预测输出.由于贝叶斯支持向量机可以有效反映随机影响及其传递,可以克服数据不确定性和相关性的影响,因此基于贝叶斯支持向量机预测效果更加符合实际.实例表明利用贝叶斯支持向量机预测高科技企业发展趋势与实际发展趋势接近,可以克服数据相关性、不确定性和非线性对信息模型的影响,具有较高的预测精度和预测鲁棒性.Abstract: To solve the uncertainty, nonlinear and coupling problem of statistical data, Bayes support vector machine(BSVM) was proposed to predict their development trend. Herein, the uncertainty of data was described as BSVM weights with Gauss distribution. Based on the prior probability and Bayes theory, the parameters evaluation of BSVM was transformed into parameters optimization of posterior distibution, which can be obtained by the prior probability and Bayes theory. The nonzero vector as correlative support vector machine was selected, and the multiple dimension prediction model based on time serials and its parameters distribution were established. Considering the input of BSVM as random variable during every iterative process, the output of BSVM can be obtained with uncertainty transferring. Since BSVM can describe the influence of random variables and its tranferring, it can overcome the uncertainty and dependence influence and the prediction results approach to the real condition. Application indicates that the prediction of high-tech enterprise development based on BSVM can approach the actual condition with high precision and robust.
-
Key words:
- Bayes /
- support vector machine /
- prediction model
-
[1] Galton F J.Regression towards mediocrity in hereditarstature [J].Journal of Anthropological Inst,1885,15:246-263 [2] 王惠文,吴载斌,孟洁.偏最小二乘回归的线性和非线性方法[M].北京:国防工业出版社,2006 Wang Huiwen,Wu Zaibin,Meng Jie.Partial least-squares regression of linearity and nonlinearity[M].Beijing:National Defense Industry Press,2006(in Chinese) [3] Wold S,Martens H,Wold H.The multivariate calibration problem in chemistry solved by the PLS method[M].Heidelberg:Springer-Verlag,1983 [4] Hsieh W W,Benyang T.Applying neural network models to prediction and data analysis in meteorology and oceanography .NASA NNX09AB39G,1998 [5] Vapink V.The nature of statistical leaning theory[M].New York:Springer-Verlag,1995 [6] 罗雪晖,李霞,张基宏.支持向量机及其应用研究[J].深圳大学学报,2003,20(3):40-46 Luo Xuehui,Li Xia,Zhang Jihong.Support vector machine and its application[J].Journal of Shenzhen University,2003,20(3):40-46(in Chinese) [7] 何莎.高校孵化器发展历程与贡献 .北京航空航天大学科技园统计报告,2008 He Sha.The development and contribution of university science and technology park .Statistical report of Beijing University of Aeonautics and Astronautics Science Park,2008(in Chinese) -
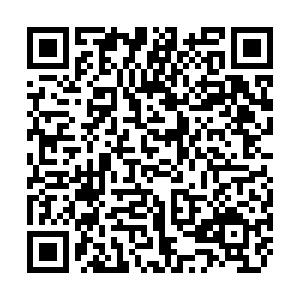
计量
- 文章访问数: 3006
- HTML全文浏览量: 226
- PDF下载量: 2426
- 被引次数: 0