Video quality assessing model based on single image quality with different weights
-
摘要: 利用人眼视觉特性与视频序列时空相关特性,提出了基于视频序列内单帧图像质量加权的视频质量评价模型.其中,单帧图像质量利用峰值信噪比和结构相似性度量作为图像质量的描述参数,采用神经网络(NN,Neural Network)与支持向量机(SVM,Support Vector Machines)建立图像质量评价模型;视频序列质量由序列内单帧图像质量加权衡量,加权因子描述了视频序列内运动及场景变化的剧烈程度.仿真实验结果表明,该模型的输出能有效地反映图像的主观质量.模型预测出的单帧图像质量和视频序列质量的单调性相比PSNR分别提高7.42%和10.47%,均方根误差相比则提高了36.06%和10.48%.Abstract: Concerning HVS (human visual system) physiological characteristic with the temporal-spatio correlations of video sequence, a video quality assessing model was proposed based on single image quality with different weights. Single image quality was assessed by using NN (neural network) and SVM (support vector machine) with PSNR (peak signal to noise ratio) and SSIM (structure similarity) as two indexes describing image quality. Video quality was assessed by using the quality of each frames in the video sequence with different weights. Those weights described motion and scene changes in the video. The monotonicity of the method for images & video is 7.42% and 10.47% higher than that of PSNR and RMSE (root mean square error) is 35.90% and 10.48% higher than that of PSNR at least. The results from simulation experiments show the model is valid.
-
Key words:
- support vector machines /
- neural networking /
- video quality /
- scene changes
-
[1] Watson A B, Solomon J A. Model of visual contrast gain control and pattern masking [J]. Journal of Optical Society of America, 1997,14(9):2379-2391[2] Vanden C J, Branden Lambrecht, Costantini D M, et al. Quality assessment of motion rendition in video coding [J]. IEEE Trans Circuits and Systems for Video Tech, 1999,9(5):766-782[3] 佟雨兵,胡薇薇,杨东凯,等.视频图像质量评价综述[J].计算机辅助设计与图形学学报,2006,18(5):1-7 Tong Yubing, Hu Weiwei, Yang Dongkai, et al. Video quality assessment methods overview[J]. Journal of CAD & Computer Graphics, 2006,18(5):1-7 (in Chinese)[4] Zhou Wang, Alan C Bovik, Eero P Simoncelli. Structural approached to image quality assessment[M]. 2nd edition, In Handbook of Image and Video Processing. New York:Academic Press, 2005[5] Vladimir N Vapnik. An overview of statistical learning theory [J]. IEEE Transactions on Neural Networks, 1999,10(5):988-999[6] JPEG DATABASE . 2002-04-15 . [7] RRNR-TV Group TEST PLAN . Draft version 1.7, 2004-06-21 . -
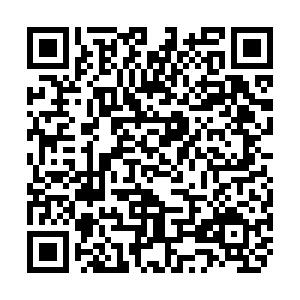
计量
- 文章访问数: 2852
- HTML全文浏览量: 47
- PDF下载量: 4293
- 被引次数: 0